Chartered Financial Data Scientist
Become a professional expert in Data Science
Contact
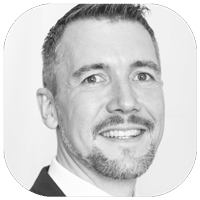
Stefan Schummer
Start date
Start 21. November 2024
Downloads
Data is the new oil
Creating value in financial services depends critically on the ability to draw reliable inferences from large quantities of heterogeneous data. Against this background, the Chartered Financial Data Science programme will provide you with essential tools and techniques for processing, analysing, and visualizing such information. You will learn to analyse time series and cross-sectional data in order to make forecasts, assess risks, perform simulations, and assign probabilities to possible events. Each of the relevant methods and modelling strategies is explained by one or more specific examples using real-world financial data. A comprehensive introduction to Python, one of the most popular programming languages for data science, will enable you to implement, extend and vary the techniques learned in accordance with the individual aims and needs.
You will significally enhance your abilities and career prospects in six distinct ways:
- Understand the implications of the gradual shift from the assumption based decision making of the 20th century to the evidence-based, data-driven decision making of the 21st century.
- Learn to critically assess the information value of a variety of different data sets based on the data source and scientific characteristics.
- Learn to understand asset management as a data–analysis–decision–data process, including general knowledge of the most useful statistical procedures for explaining the variation of asset prices.
- Enjoy a practical session of training in the currently most popular programming language of Financial Data Science: Python.
- Will be introduced into the world of Big Data, machine learning, and deep learning methods to source insights from these data riches.
- Learn how to visualize and communicate valuable insights gained through Financial Data Science.
After passing the exam and conducting a three month project work, successful candidates are granted the title
CFDS® – Chartered Financial Data Scientist
Content
Financial Statistic
Maschine Learning & AI
1. Introduction: Data Science
2. Exploratory Data Analysis
3. Multiple Linear Regression and Related Topics
4. Models with Binary Dependent Variables
5. Time-Varying Volatility and the Likelihood of Extreme Loss Events
6. Smoothed Bootstrap
7. Statistics with Python
1. Introduction to Machine Learning
2. Introduction to Python and ML Libraries
3. Supervised Learning and Logistic Regression
4. Unsupervised Learning and K-Means
5. Neural Networks and Deep Learning
6. Convolutional Neural Networks
7. Recurrent Neural Networks and Long Short Term Memory
8. Natural Language Processing
9. Reinforcement Learning
10. Data Centric AI
11. AI Canvas
a) Introduction to Financial Data Science
b) Exploring and Analysing Data
c) Data & Asset Management: does the asset create data or is independent data the asset?
d) The Science of Data
e) Understanding Asset Management from a financial data science perspective
f) Statistical Analysis of asset price variation
g) Python for Financial Data Science
h) Big Data Storage and Retrieval
i) Machine Learning
j) Deep Learning
k) Data Visualization and Communication of Outcomes
Timestructure
The CFDS program consists of 2 in-class blocks. One Kick off at the beginning and a presentation at the end. In between you will have 15 live webinars as well as video material for your self-study. (Study time: approx. 145 hours).
This knowledge in financial data science will be subject to a two hour multiple choice exam.
All students passing that exam will then start a three months project work, that means analysing a real or fictive data set using Python. The results of the project work will be presented in a two-day closing session.
Target group
Managers and employees from the following segments
- Data Analytics
- Data Management
- Risk Management
- Marketing/Sales
- Trading
- Compliance/Regulation
- IT
- interface product management/ project management and IT
our CFDS - Experts
Dr. Sikandar Siddiqui
Sikandar Siddiqui is an economist and financial analyst specializing in asset valuation, risk management, and applied econometrics. Having gathered more than twenty years of professional experience in the fields of finance and management consulting, he currently serves as Head of Quantitative Methods at Deloitte Audit Analytics Germany.
Sikandar has authored or co-authored several articles on economic, statistical and financial issues. He holds a doctoral degree in economics from the University of Konstanz and has qualified as a CFA Charterholder.
Prof. Dr. Natalie Packham
Natalie Packham is Professor of Mathematics and Statistics at Berlin School of Economics and Law and Principal Researcher within the International Research Training Group “High Dimensional Nonstationary Time Series” (IRTG 1792) at Humboldt University Berlin. Natalie has several years of industry experience as a front office software engineer at an investment bank, and is frequently involved in industry-related research and consulting projects. Her research expertise includes Mathematical Finance, Financial Risk Management and Computational Finance, and her academic work has been published in Mathematical Finance, Finance & Stochastics, Quantitative Finance, Journal of Applied Probability and many other academic journals. She is associate editor of “Methodology and Computing in Applied Probability” and “Digital Finance” and co-chair of the GARP Research Fellowship Advisory Board. Natalie holds an M.Sc. in Computer Science from the University of Bonn, a Master’s degree in Banking & Finance from Frankfurt School, and a Ph.D. in Quantitative Finance from Frankfurt School.
Prof. Dr. Christina Erlwein-Sayer
Prof. Dr. Christina Erlwein-Sayer is Professor of Financial Mathematics and Statistics at Hochschule für Technik und Wirtschaft (HTW) Berlin. Her research interests lie in Financial Mathematics and Risk Management with a focus on statistical learning in finance, financial modelling, portfolio optimisation, and risk management with sentiment analysis. Christina holds a Master’s degree in Business Mathematics from University of Trier and completed her Ph.D. in Mathematics at Brunel University, London, UK in 2008. She has several years of industry experience in Financial Mathematics as a researcher and consultant at Fraunhofer ITWM, Kaiserslautern, Germany. Prior to joining HTW in 2019, she was a quantitative analyst and senior researcher at OptiRisk Systems London, UK.
Stimmen unserer Absolventen
Sie haben Fragen?
Sie möchten mehr über das DVFA-Programm „Chartered Financial Data Scientist“ wissen.
Herr Schummer freut sich auf Ihren Anruf: 069-26 48 48-121
Oder schreiben Sie eine kurze Nachricht an ssc@dvfa.de